Data Warehouse Examples: Explained
In today’s data-driven world, organizations are constantly seeking ways to harness the power of their information assets. One of the most effective tools for managing and analyzing large volumes of data is a data warehouse. This comprehensive guide will explore various data warehouse examples, concepts, and use cases, providing you with a deep understanding of how data warehouses can transform an organization’s decision-making processes.
As we delve into the world of data warehouses, we’ll examine their fundamental components, explore different types of implementations, and showcase real-world examples across various industries. Whether you’re a business leader looking to leverage data for strategic advantage, an IT professional planning a data warehouse implementation, or simply curious about how large-scale data management works, this guide will offer valuable insights into the power and potential of data warehouses.
What is a Data Warehouse?
A data warehouse is a centralized repository that stores large volumes of structured and semi-structured data from various sources within an organization. Unlike traditional databases designed for day-to-day transactions, data warehouses are specifically engineered for query and analysis, playing a crucial role in business intelligence (BI) and analytics. They enable organizations to make data-driven decisions based on comprehensive historical and current data analysis.
To truly grasp the concept, let’s break down the key characteristics that define a data warehouse:
Subject-oriented: Data warehouses focus on specific business areas or subjects, such as sales, inventory, or customer behavior. This orientation allows for deep, targeted analysis of particular aspects of the business.
Integrated: One of the most powerful features of a data warehouse is its ability to combine data from multiple, often disparate sources into a consistent format. This integration process resolves inconsistencies in data formats, naming conventions, and coding structures, providing a unified view of the organization’s data.
Time-variant: Unlike operational databases that mainly store current data, data warehouses maintain historical data over an extended period. This time-based data storage enables trend analysis, forecasting, and the ability to track changes over time, which is crucial for strategic decision-making.
Non-volatile: Once data is loaded into a data warehouse, it remains stable and doesn’t change. This stability ensures consistent results for queries and reports, even as new data is added regularly.
To illustrate these concepts, let’s consider a practical example. Imagine a retail chain with hundreds of stores across the country. Each store has its own point-of-sale system, the company runs an e-commerce website, and there’s a separate customer relationship management (CRM) system. A data warehouse would integrate all these data sources, allowing the company to analyze:
- Sales trends across all channels over time
- Customer behavior patterns both in-store and online
- Inventory levels and movement across the entire supply chain
- Effectiveness of marketing campaigns on different customer segments
By centralizing and structuring this data, the retailer can gain insights that would be impossible to derive from the individual systems alone.
Key Components of a Data Warehouse
Understanding the key components of a data warehouse is essential for grasping how these systems function and deliver value. Let’s explore each component in detail:
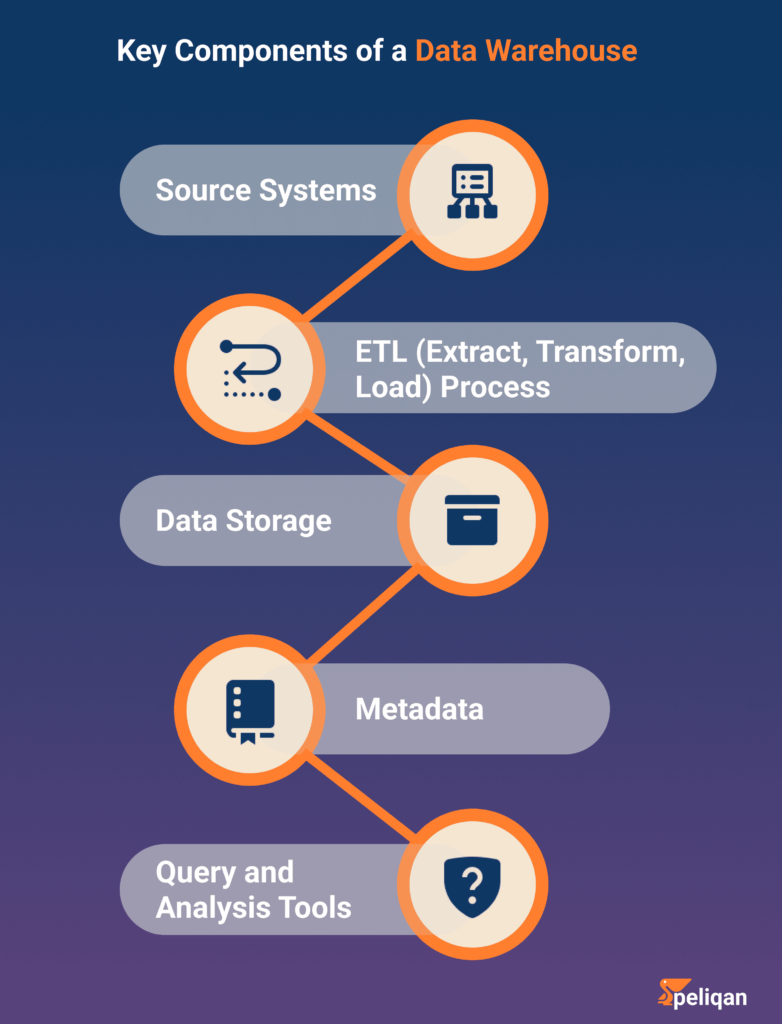
a) Source Systems:
These are the various operational databases and external data sources that feed into the data warehouse. In a typical organization, source systems might include:
- Transactional databases (e.g., sales, inventory, finance)
- Customer Relationship Management (CRM) systems
- Enterprise Resource Planning (ERP) systems
- External data sources (e.g., market research data, social media feeds)
Each of these systems generates data in its own format and structure, which leads us to the next crucial component.
b) ETL (Extract, Transform, Load) Process:
The ETL process is the backbone of data warehousing. It involves:
Extract: Data is extracted from various source systems. This can be a complex process, especially when dealing with legacy systems or unstructured data sources.
Transform: The extracted data is cleaned, standardized, and transformed to fit the data warehouse schema. This step might involve:
- Cleaning data to remove errors or inconsistencies
- Resolving naming conflicts (e.g., ensuring “customer_id” in one system matches “cust_number” in another)
- Converting data types or units of measurement for consistency
- Aggregating data to the appropriate level of detail
Load: The transformed data is loaded into the data warehouse. This can be done in batches (e.g., nightly updates) or in real-time, depending on the organization’s needs.
c) Data Storage:
This is the actual repository where the processed data is stored. Modern data warehouses often use columnar storage techniques for efficient querying of large datasets. The data is typically organized using dimensional modeling techniques, which we’ll explore in more detail later.
d) Metadata:
Metadata is essentially “data about data.” It includes information about:
- The structure of tables and relationships between them
- Data lineage (where the data came from and how it was transformed)
- Update frequencies and schedules
- Access rights and security policies
Good metadata management is crucial for maintaining the integrity and usability of the data warehouse.
e) Query and Analysis Tools:
These are the software applications that allow users to access, analyze, and visualize the data stored in the warehouse. They range from simple reporting tools to advanced analytics platforms and can include:
- SQL clients for direct querying
- Business Intelligence (BI) platforms like Tableau or Power BI
- Statistical analysis tools like R or Python libraries
- Machine learning platforms for predictive analytics
Types of Data Warehouses
Data warehouses come in various types, each suited to different organizational needs and structures. Understanding these types can help in choosing the right approach for a specific business context. Let’s explore the main types of data warehouses:
a) Enterprise Data Warehouse (EDW):
An Enterprise Data Warehouse is a centralized warehouse that provides a single source of truth for the entire organization. It integrates data from all departments and functions, offering a comprehensive view of the business.
Key characteristics:
- Covers all major subject areas of the organization
- Highly structured and integrated data
- Supports cross-functional analysis and reporting
- Typically requires significant investment and long implementation time
b) Operational Data Store (ODS):
An Operational Data Store is a type of data warehouse that stores current, detailed data for operational reporting. It serves as an intermediate step between operational systems and the data warehouse, providing near real-time data for operational decision-making.
Key characteristics:
- Focuses on current data rather than historical records
- Updated frequently, often in real-time or near real-time
- Supports day-to-day operations and tactical decision-making
- Often used as a staging area before data is transferred to the main data warehouse
c) Data Mart:
A data mart is a subset of a data warehouse that focuses on a specific business line or department. Data marts can be dependent (derived from an existing data warehouse) or independent (sourced directly from operational systems).
Key characteristics:
- Focused on a specific subject area or department
- Faster to implement and easier to manage than full-scale data warehouses
- Provides more control to individual departments
- Can be used as building blocks for a larger data warehouse strategy
d) Cloud Data Warehouse:
A cloud data warehouse is hosted on a cloud computing platform, offering scalability, flexibility, and often reduced infrastructure costs compared to on-premises solutions.
Key characteristics:
- Scalable storage and computing resources
- Pay-as-you-go pricing models
- Built-in security and compliance features
- Easy integration with other cloud services
e) Real-time Data Warehouse:
A real-time data warehouse can process and make data available for analysis in real-time or near-real-time, enabling immediate insights and actions based on the most current data.
Key characteristics:
- Continuous data ingestion and processing
- Support for streaming data sources
- Low-latency querying capabilities
- Often uses in-memory processing for speed
These various
types of data warehouses demonstrate the flexibility of the concept to adapt to different business needs, data volumes, and analysis requirements. As we continue our exploration of data warehouse examples, we’ll see how these different types are applied in various industries and use cases.
Industry-Specific Data Warehouse Examples
Different industries have unique data warehousing needs. Let’s explore some industry-specific data warehouse examples to understand how these systems are tailored to meet various business requirements.
Data Warehouse Example: Retail Industry
Use Case: Customer 360 View and Personalization
In the retail sector, data warehouses are crucial for creating a comprehensive view of customers across multiple channels. A typical retail data warehouse integrates:
- Point-of-sale data from physical stores
- E-commerce transactions
- Customer service interactions
- Marketing campaign data
- Inventory and supply chain information
This integration enables retailers to:
- Develop personalized product recommendations
- Optimize pricing and promotions based on customer segments
- Improve inventory management across channels
- Enhance the overall customer experience
Real-world example:
Target Corporation, one of the largest retailers in the United States, uses a sophisticated data warehouse to power its analytics and decision-making. Their system, known as the “Guest Data Platform,” integrates data from various sources to create a unified view of each customer. This has enabled Target to:
- Implement highly successful personalized marketing campaigns
- Optimize store layouts based on customer behavior analysis
- Improve inventory management, reducing stockouts and overstocks
- Enhance their online and mobile shopping experiences
Data Warehouse Example: Healthcare Industry
Use Case: Population Health Management and Operational Efficiency
Healthcare organizations use data warehouses to analyze patient data, improve care quality, and optimize operations. A healthcare data warehouse typically includes:
- Electronic Health Records (EHR)
- Claims and billing data
- Pharmacy data
- Laboratory results
- Medical imaging data
This comprehensive data integration allows healthcare providers to:
- Identify high-risk patients for preventive care
- Analyze treatment effectiveness across different demographics
- Optimize resource allocation based on population health trends
- Improve patient outcomes through data-driven decision making
Real-world example:
Kaiser Permanente, one of the largest healthcare providers in the U.S., implemented a massive data warehouse called “HealthConnect.” This system integrates data from millions of patient records across all their facilities. With HealthConnect, Kaiser Permanente has been able to:
- Reduce hospital stays by identifying at-risk patients earlier
- Improve chronic disease management through better tracking and intervention
- Enhance research capabilities, leading to improved treatment protocols
- Streamline operations and reduce administrative costs
Data Warehouse Example: Financial Services Industry
Use Case: Risk Management and Fraud Detection
Financial institutions leverage data warehouses for a variety of critical functions, including risk assessment, fraud detection, and regulatory compliance. A typical financial services data warehouse incorporates:
- Transaction data from various banking channels
- Customer account information
- Credit scoring data
- Market data feeds
- Regulatory reporting data
This integrated data allows financial institutions to:
- Perform real-time risk analysis on trading positions
- Generate regulatory compliance reports
- Detect fraudulent activities through pattern recognition
- Personalize financial products and services
Real-world example:
JPMorgan Chase, one of the world’s largest banks, utilizes a sophisticated data warehouse infrastructure to manage risk and enhance customer services. Their system, which processes petabytes of data daily, enables:
- Real-time fraud detection across millions of transactions
- Comprehensive risk management across diverse financial products
- Personalized banking experiences for retail and institutional clients
- Advanced analytics for investment strategies and market analysis
Data Warehouse Example: Manufacturing Industry
Use Case: Supply Chain Optimization and Predictive Maintenance
In manufacturing, data warehouses play a crucial role in optimizing production processes and managing complex supply chains. A manufacturing data warehouse typically includes:
- Production line data from IoT sensors
- Quality control measurements
- Inventory and supply chain data
- Customer order information
- Equipment maintenance records
This integrated data allows manufacturers to:
- Optimize inventory levels across the supply chain
- Predict maintenance needs for manufacturing equipment
- Analyze quality control data to improve product reliability
- Enhance production scheduling and resource allocation
Real-world example:
Siemens, a global leader in industrial manufacturing, implemented a company-wide data warehouse called “One Siemens.” This system integrates data from various business units and manufacturing facilities worldwide. With One Siemens, the company has achieved:
- Improved forecasting and demand planning across its diverse product lines
- Enhanced predictive maintenance capabilities, reducing downtime in its factories
- Optimized global supply chain management
- Accelerated product development through better data sharing and analysis
Data Warehouse Example: Telecommunications Industry
Use Case: Network Performance and Customer Experience Management
Telecom companies use data warehouses to analyze vast amounts of network data and customer usage patterns. A telecom data warehouse typically includes:
- Network performance data
- Call Detail Records (CDRs)
- Customer subscription and billing information
- Service quality metrics
- Social media and customer feedback data
This integrated data enables telecom providers to:
- Identify areas for network infrastructure improvements
- Develop personalized service plans based on usage patterns
- Predict and prevent customer churn through advanced analytics
- Optimize network resources in real-time
Real-world example:
Verizon, one of the largest telecommunications companies in the world, utilizes a massive data warehouse to manage its network and improve customer services. Their system processes billions of records daily, allowing Verizon to:
- Optimize network performance in real-time, reducing outages and improving service quality
- Personalize customer offers based on usage patterns and preferences
- Detect and prevent fraud more effectively
- Improve customer service through predictive analytics and proactive issue resolution
Best Data Warehouse Tool: Peliqan
Peliqan, an all-in-one data platform designed to simplify and streamline the entire data management process. Peliqan stands out in the data warehousing landscape by offering a comprehensive suite of tools and features that cater to businesses of all sizes, from startups to large enterprises.
What sets Peliqan apart is its ability to handle the entire data lifecycle – from ingestion to activation – without the need for a dedicated data engineering team.
Key Features of Peliqan:
Comprehensive Data Integration:
- Connect to over 100 SaaS applications, databases, and file sources
- Built-in data warehouse option or integration with existing solutions like Snowflake, BigQuery, Redshift, or SQL Server
- Automated ETL data pipelines requiring zero maintenance
Flexible Data Transformation:
- SQL-based data modeling and transformation
- Low-code Python scripting for advanced transformations
- Spreadsheet-like interface for business users to explore and edit data
Advanced Analytics and AI Integration:
- AI-assisted SQL query writing for quick insights
- Integration with popular BI tools
- One-click deployment of data tools like Metabase, Jupyter notebooks, and Apache Superset
Data Activation and Sharing:
- Reverse ETL capabilities for syncing data back to business applications
- API endpoint publishing for data products and ML models
- Custom alerting and report distribution
Data Governance and Lineage:
- Automatic detection of table and column lineage
- Built-in data catalog for metadata management
Low-Code Development:
- Build data apps, APIs, and syncs with minimal coding
- Combine SQL, low-code Python, and AI for powerful data solutions
Real-World Applications of Peliqan:
- Startups and Scale-ups: Peliqan enables fast-growing companies to quickly set up a robust data infrastructure without the need for a large data team. This allows them to make data-driven decisions from day one and scale their data operations as they grow.
- Business Teams: With its user-friendly interface and low-code capabilities, Peliqan empowers business users to explore data, create reports, and set up data workflows without heavy reliance on IT departments.
- IT Service Companies: Peliqan provides IT service providers with a versatile platform to manage multiple clients’ data needs efficiently, from data integration to advanced analytics and reporting.
- Enterprise Data Democratization: Large organizations can use Peliqan to democratize data access across departments, ensuring that everyone has the tools they need to work with data effectively.
By offering a unified platform for data warehousing, ETL, analytics, and data activation, Peliqan addresses many of the challenges we’ve discussed earlier in this post. It simplifies data integration, reduces the technical barriers to data analysis, and provides the flexibility needed to adapt to changing business requirements.
Conclusion
Data warehouses have become indispensable tools for organizations seeking to leverage their data assets for competitive advantage. From retail to healthcare, finance to manufacturing, the examples we’ve explored demonstrate the versatility and power of data warehouses in driving business intelligence and decision-making.
As data continues to grow in volume, variety, and velocity, the role of data warehouses in helping organizations make sense of this information will only become more critical. The future of data warehousing looks bright, with advancements in cloud computing, artificial intelligence, and real-time processing promising even greater capabilities and insights.
Whether you’re considering implementing your first data warehouse or looking to upgrade an existing system, remember that success lies not just in the technology chosen, but in aligning the warehouse design with your specific business needs and goals. By understanding these data warehouse examples and concepts, you’re well-equipped to embark on your data warehousing journey, transforming raw data into actionable insights that can propel your business forward in the data-driven economy.
As you move forward, consider starting small with a focused data mart or cloud-based solution, and gradually expand as you gain experience and demonstrate value. Remember that a successful data warehouse implementation is an ongoing journey of continuous improvement and adaptation to evolving business needs and technological advancements.
FAQ’s
1. Is Excel an example of a data warehouse?
No, Excel is not a data warehouse. While Excel can be used for data analysis and storage of small datasets, it lacks the scalability, integration capabilities, and advanced features of a true data warehouse. Data warehouses are designed to handle much larger volumes of data from multiple sources and provide more sophisticated analysis and reporting capabilities.
2. What are the three types of data warehouses?
The three main types of data warehouses are:
- Enterprise Data Warehouse (EDW): A centralized warehouse for all of an organization’s data.
- Operational Data Store (ODS): A warehouse that stores current, detailed data for operational reporting.
- Data Mart: A subset of a data warehouse that focuses on a specific business line or department.
Additionally, there are other types such as Cloud Data Warehouses and Real-time Data Warehouses, which we discussed earlier in this article.
3. What is data warehousing with its application and example?
Data warehousing is the process of collecting, storing, and managing data from various sources to support business intelligence activities. Applications include business reporting, analytics, and decision support. An example is a retail company using a data warehouse to integrate sales data from stores, online platforms, and mobile apps to analyze customer behavior, optimize inventory, and personalize marketing campaigns.
4. Is SQL a data warehouse?
No, SQL (Structured Query Language) is not a data warehouse. SQL is a programming language used for managing and querying relational databases. While SQL is often used to interact with data warehouses, it is a tool rather than a data warehouse itself. Data warehouses may use SQL-based systems for data storage and retrieval, but they encompass much more than just the query language.
5. What are examples of a data warehouse?
Top data warehouse examples include:
- Peliqan
- Amazon Redshift
- Google BigQuery
- Snowflake
- Microsoft Azure Synapse Analytics
- Oracle Autonomous Data Warehouse
- Teradata
- IBM Db2 Warehouse
6. What is ETL in data warehousing?
ETL stands for Extract, Transform, Load. It is a crucial process in data warehousing that involves:
- Extract: Collecting data from various source systems
- Transform: Cleaning, validating, and converting the data into a consistent format
- Load: Inserting the transformed data into the data warehouse
ETL is essential for ensuring that data in the warehouse is accurate, consistent, and ready for analysis. It helps in integrating data from different sources and formats into a unified structure within the data warehouse.