Data Warehouse Tools
In today’s data-driven world, businesses rely heavily on data warehouses to store and analyze large historical datasets from multiple sources. A well-designed data warehouse architecture enables organizations to transform raw data into actionable insights, supporting informed decision-making. With numerous data warehouse solutions available, choosing the right tool can be a daunting task.
This comprehensive guide explores the top 15 data warehouse examples and best data warehouse tools, highlighting their key features and considerations to help you make an informed choice. Before getting into the list, let’s understand some basics.
What Are Data Warehouses?
A data warehouse is a centralized repository designed to store and manage large volumes of historical data from various sources within an organization. Optimized for data analysis, warehouses facilitate comprehensive exploration of trends and patterns, enabling businesses to gain deeper insights and make data-driven strategic decisions.
Data warehouses offer significant advantages over traditional databases for large-scale historical data analysis. They provide faster processing, improved data quality, and deeper insights, empowering businesses to:
- Perform advanced business intelligence and reporting
- Conduct data mining and analytics
- Develop decision support systems
- Enhance customer relationship management (CRM)
- Enable financial analysis and forecasting
Enterprise Data Warehouse (EDW) Market size is set to grow by USD 39.23 billion from 2024-2028, Data explosion across industries boost the market. This creates the sense of urgency in leveraging the modern data warehouse tools to stay competitive.
Data Warehouse Tools: What to Look for
When evaluating data warehouse tools, consider the following key factors:
- Scalability: Ensure the tool can handle your current data volume and scale as your needs grow. Look for solutions that offer elastic scaling options, allowing you to adjust resources based on demand without significant downtime or performance issues.
- Performance: Look for fast query processing and data loading capabilities. Evaluate tools based on their ability to handle complex queries efficiently and support concurrent users without compromising speed.
- Ease of use: Consider the learning curve and user-friendliness of the interface. A good data warehouse tool should offer intuitive dashboards, drag-and-drop functionality for query building, and clear documentation to help users get up to speed quickly.
- Integration capabilities: Check compatibility with your existing data sources and analytics tools. The ideal solution should offer a wide range of pre-built connectors and support for standard protocols to ensure seamless data flow across your entire data ecosystem.
- Security features: Evaluate data encryption, access controls, and compliance certifications. Look for tools that offer end-to-end encryption, role-based access control, and compliance with industry standards such as GDPR, NIS2, or SOC 2.
- Cost-effectiveness: Analyze pricing models and total cost of ownership. Consider not just the upfront costs, but also ongoing expenses such as storage, compute resources, and potential charges for data transfer or API calls.
- Support and documentation: Assess the quality of customer support and available resources. Look for vendors that offer multiple support channels, comprehensive documentation, active user communities, and regular training sessions or webinars.
- Cloud vs. on-premises: Determine which deployment model best suits your organization’s needs. Consider factors such as data residency requirements, existing infrastructure investments, and the level of control you need over your data warehouse environment.
Data Warehouse Tools: Top 15
This blog post dives into the top 15 data warehouse solutions, highlighting their key features and considerations to help you make an informed decision as you navigate the world of data warehouse solutions. Here’re the list of top 15 data warehouse tools.
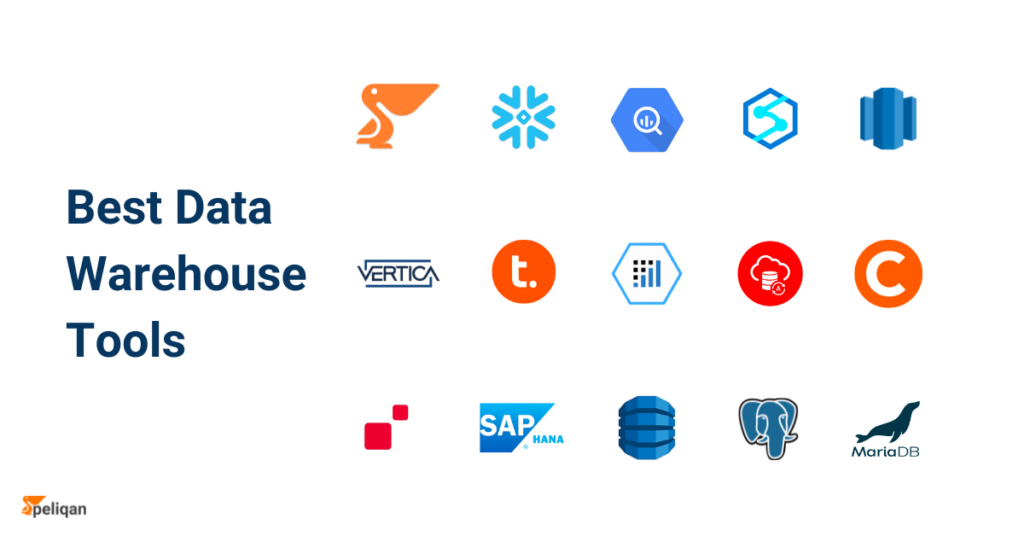
1. Peliqan.io
Peliqan.io prioritizes user-friendliness and rapid deployment for data warehousing and business intelligence. Ideal for businesses seeking a user-friendly solution to get up and running quickly.

Peliqan is an all-in-one platform for all your data needs: connect to all your business applications, ETL your data into a built-in data warehouse or Snowflake & Bigquery, use your favorite BI tool, deploy Metabase and other data tools with a single click and implement data activation such as Reverse ETL, publishing API endpoints, sending alerts, distribution of custom personalized reports, live data in Excel etc.
Features:
- Unify Data: Peliqan seamlessly connects to over 100+ data sources.
- Explore & Analyze: Dive into data with a spreadsheet-like interface and Magical SQL.
- Automate & Act: Build data apps, set alerts, and share reports in minutes.
Considerations:
- May not be suitable for very large datasets (terrabytes of data).
- Strong focus on using SQL and low-code Python, no built-in support for other scripting languages such as .NET or R
2. Snowflake
Snowflake offers a scalable, cloud-based data warehouse with elastic compute for on-demand processing power. It separates storage and compute, allowing for cost optimization.
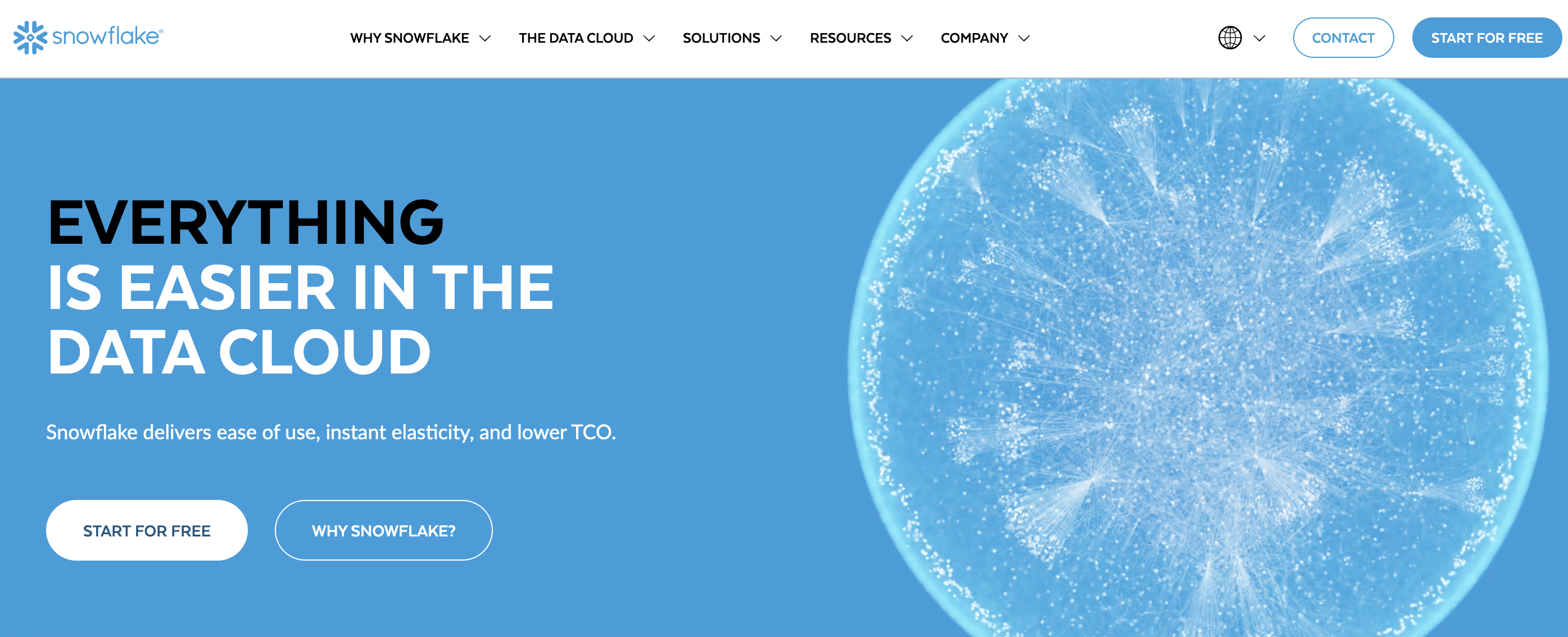
Familiar SQL query support makes data analysis accessible to existing users. Snowflake’s ultra-scalable architecture adapts to your data volume needs, making it a strong contender for organizations with growing datasets.
While Snowflake offers its own data connectors and tools, Peliqan provides an alternative approach. You can leverage Peliqan’s pre-built Snowflake connector to easily extract and transform your data. Peliqan’s user-friendly interface allows you to explore and analyze the data directly within the platform, or leverage familiar BI tools like Power BI for further visualization.
Features:
- Ultra-scalable architecture adapts to your data volume needs.
- Familiar SQL query support for data analysis by existing users.
- Cost-effective separation of storage and compute resources.
Considerations:
- Pricing structure can become complex for extensive deployments.
- Compute cost can increase rapidly.
3. Google BigQuery
Google BigQuery provides a cost-effective, serverless architecture with pay-per-use billing. It handles massive datasets with lightning-fast query speeds and boasts built-in machine learning for advanced data exploration.
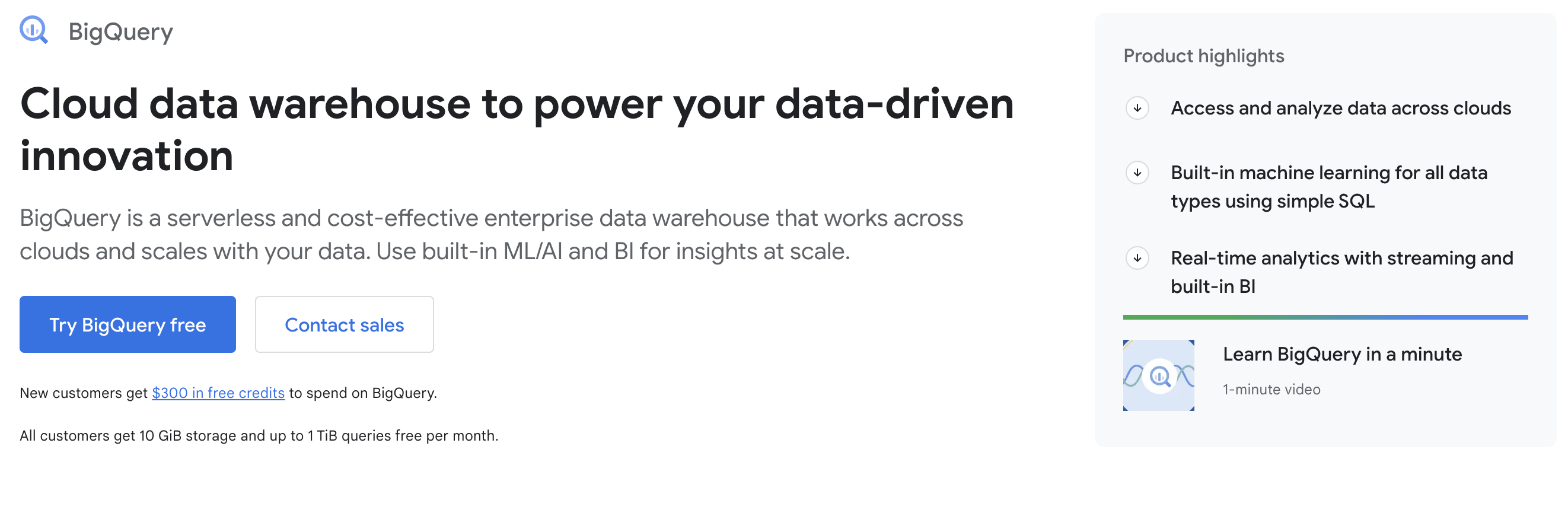
The serverless architecture eliminates infrastructure management needs, while the built-in machine learning capabilities empower you to uncover hidden patterns within your data.
Peliqan integrates seamlessly with Google BigQuery through its connector. Peliqan empowers you to import your BigQuery data, explore it in its intuitive interface, and use Magical SQL for data transformations. You can also connect your transformed data to your favorite BI tools for in-depth analysis.
Features:
- Serverless architecture eliminates infrastructure management needs.
- Handles massive datasets efficiently with blazing-fast query speeds.
- Leverages built-in machine learning for advanced data exploration.
Considerations:
- Limited data transformation capabilities compared to some options.
- Security features might require additional configuration for specific compliance needs.
4. Microsoft Azure Synapse Analytics
Azure Synapse Analytics (formerly Azure Data Warehouse) is a cloud-native data warehouse integrated with other Azure services. It unifies data warehousing and big data analytics for comprehensive insights, offering visually interactive tools for user-friendly data exploration.
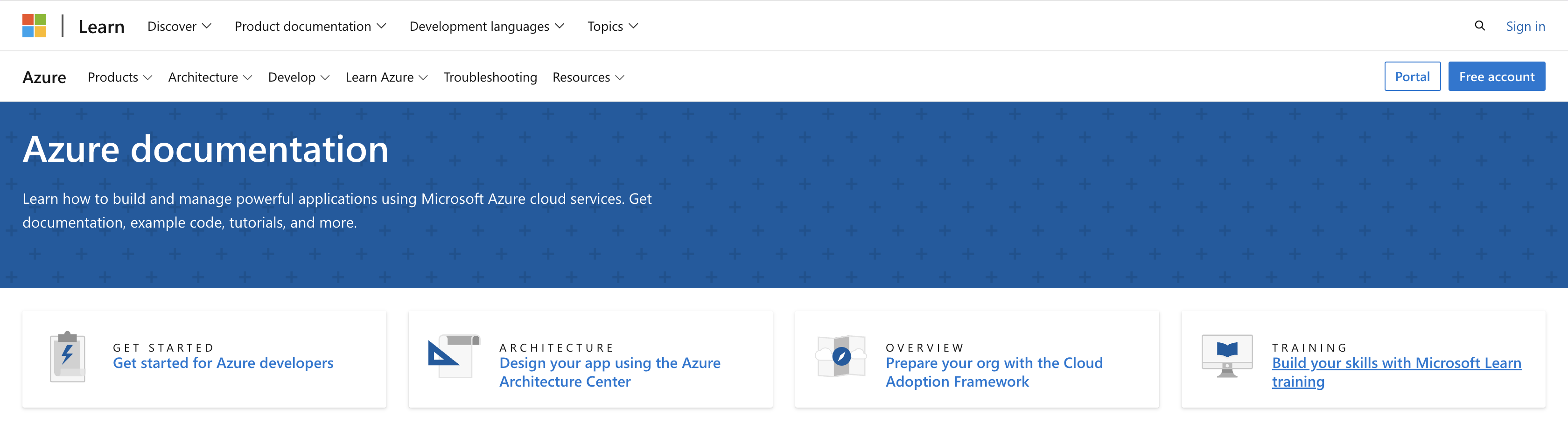
Seamless integration with other Azure services creates a unified data ecosystem, streamlining your data management processes.
Features:
- Seamless integration with other Azure services for a unified data ecosystem.
- Unites data warehousing and big data analytics for broader data exploration.
- Offers visually interactive tools for user-friendly data exploration.
Considerations:
- Steeper learning curve compared to simpler tools due to its comprehensive nature.
- Pricing can vary depending on the Azure services used in conjunction.
5. Amazon Redshift
Amazon Redshift is a scalable data warehouse service built specifically for the AWS cloud environment. It’s a cost-efficient option for analyzing large datasets stored in S3 and offers a familiar interface for AWS users.
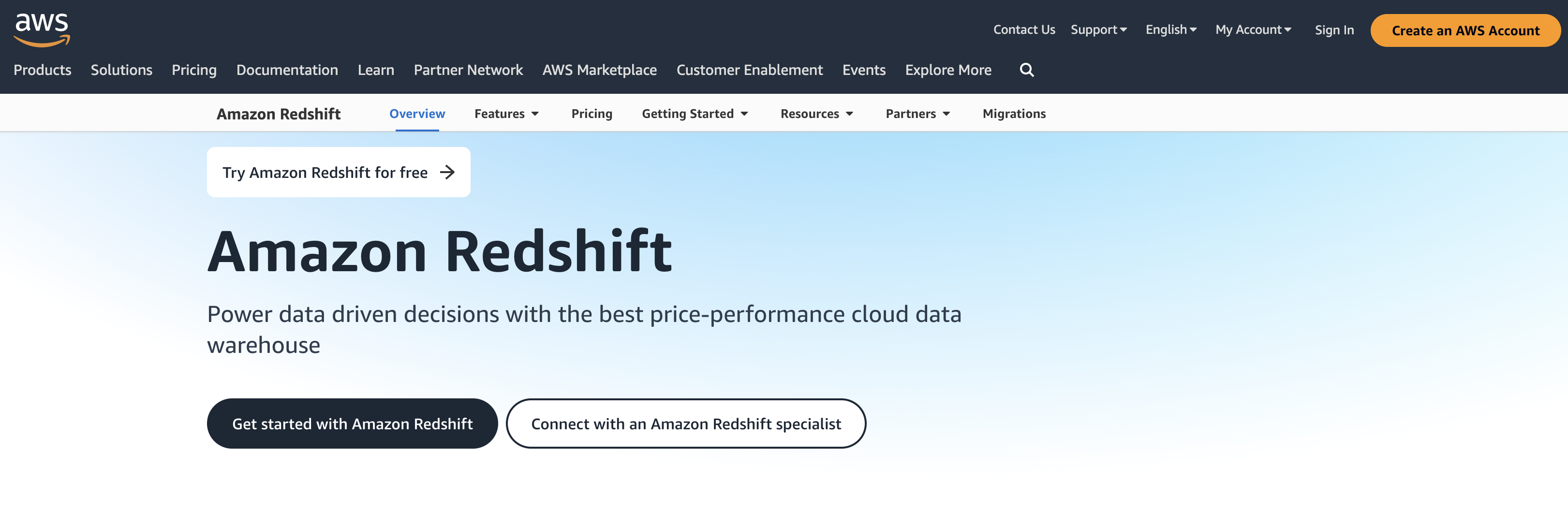
Redshift scales efficiently to handle growing data volumes, making it a valuable option for organizations already invested in the AWS cloud.
Peliqan acts as an intermediary between Redshift and your favorite data exploration tools. Its Redshift connector allows you to easily import your data and leverage Peliqan’s functionalities. Explore the data visually within Peliqan’s interface, use Magical SQL for transformations, or connect to your preferred AWS BI tools for further analysis.
Features:
- Cost-effective for analyzing large datasets stored in S3 buckets.
- Familiar interface for users comfortable with the broader AWS ecosystem.
- Scales efficiently to handle growing data volumes.
Considerations:
- May require additional configuration for optimal performance.
- Security features might require additional configuration for specific compliance needs.
6. Micro Focus Vertica
Vertica is a high-performance columnar data warehouse for complex analytical workloads. It handles large, complex datasets efficiently with advanced compression techniques, optimized for historical data querying and trend analysis.
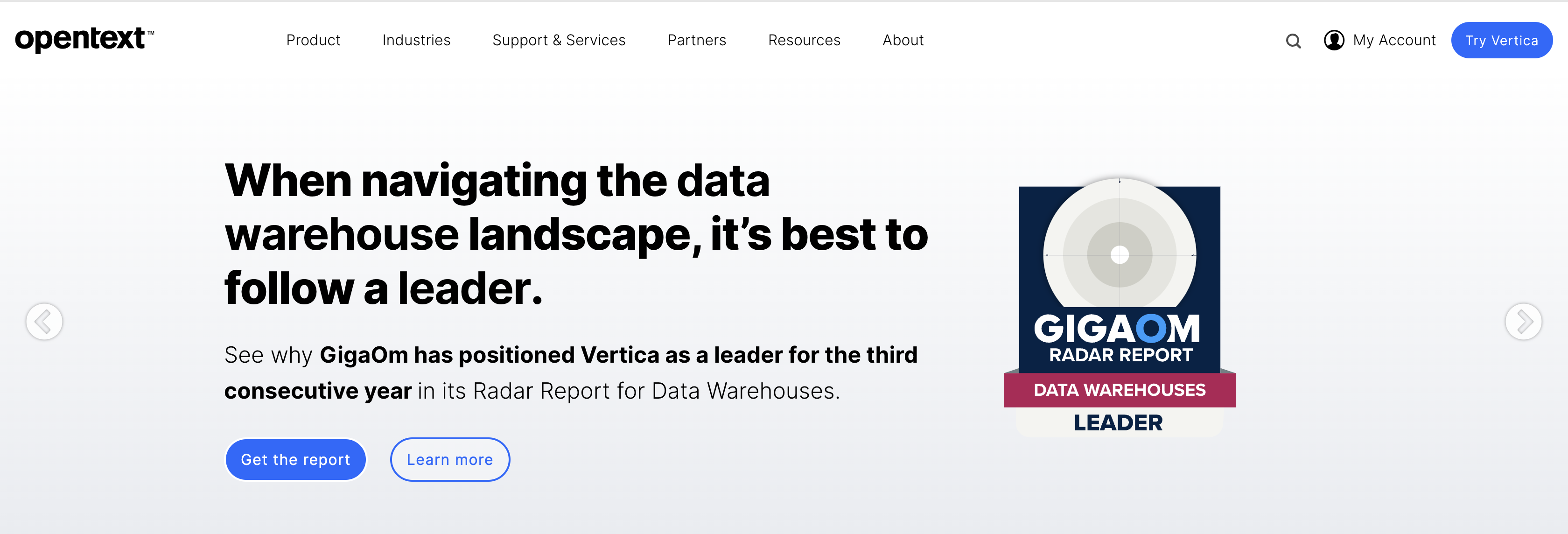
Vertica’s strength lies in its ability to efficiently query massive datasets, making it ideal for organizations with historical data that requires in-depth analysis.
Features:
- High-performance columnar storage for efficient querying of large datasets.
- Advanced compression techniques minimize storage requirements.
- Optimized for historical data querying and trend analysis.
Considerations:
- Requires significant technical expertise for setup and management.
- Not ideal for real-time data analytics due to its focus on historical data.
7. Teradata
Teradata is an enterprise-grade data warehouse solution for mission-critical deployments. It offers robust security, high availability, and a scalable architecture for massive data volumes.
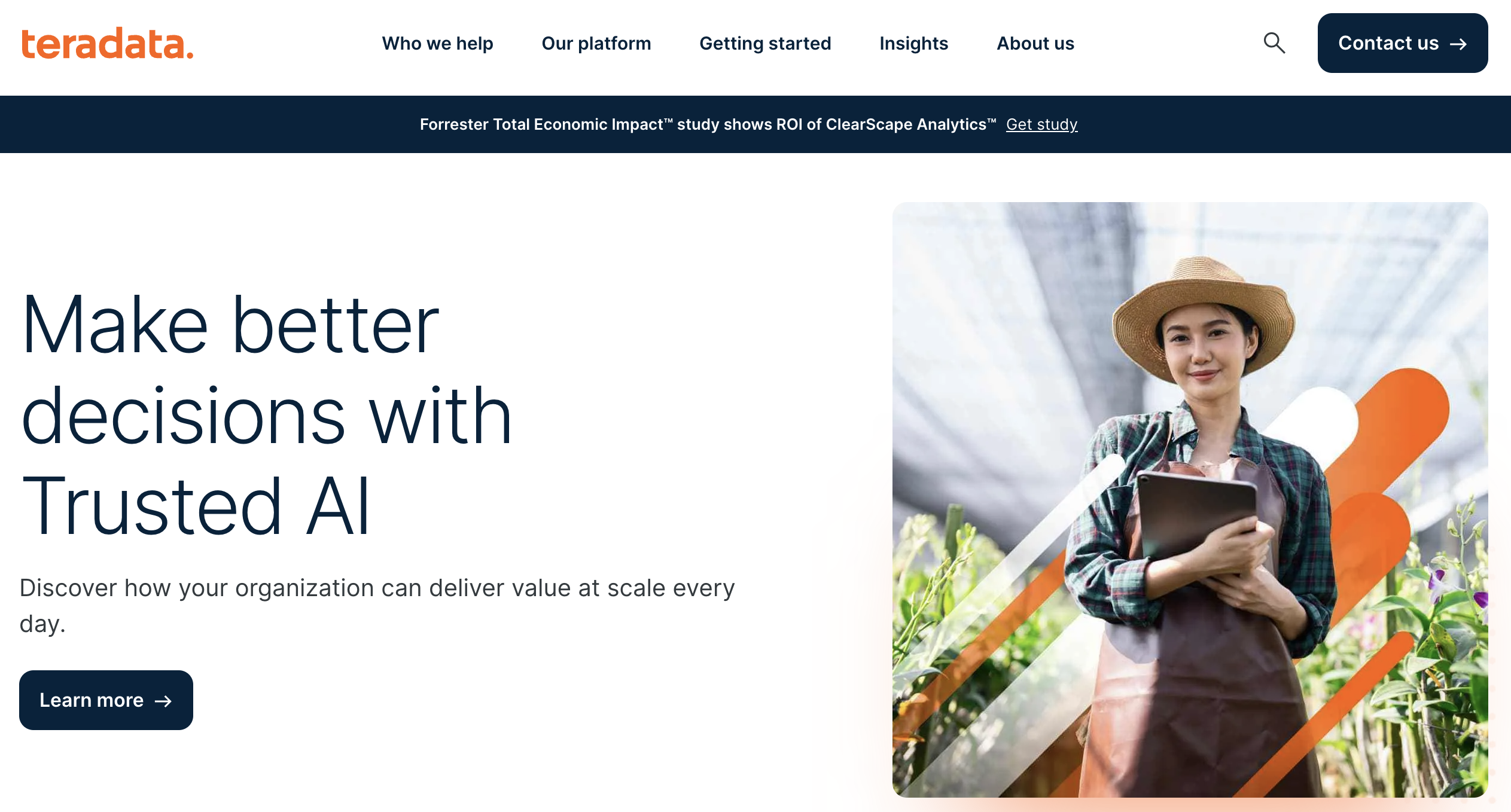
Teradata’s robust security features ensure data integrity and compliance, making it a strong choice for organizations with sensitive data.
Features:
- Robust security features ensure data integrity and compliance.
- High availability architecture guarantees minimal downtime for critical operations.
- Massively scalable architecture handles enormous data volumes efficiently.
Considerations:
- Higher cost compared to some cloud-based options.
- Complex setup and management processes require significant IT expertise.
8. IBM Db2 Warehouse
Db2 Warehouse is a secure, reliable data warehouse built for integration with IBM’s analytics ecosystem. It offers advanced data governance features and is designed for scalability and performance for demanding workloads.
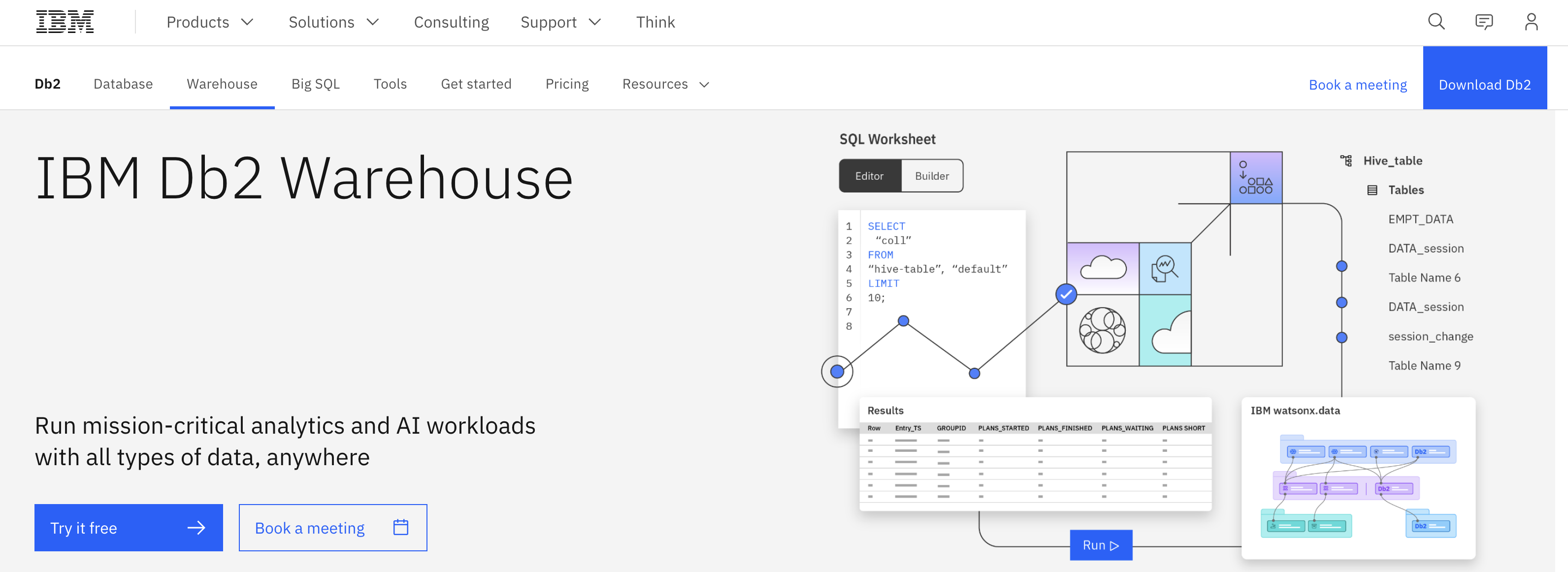
Db2 Warehouse integrates seamlessly with other IBM analytics tools, creating a unified environment for data management.
Features:
- Integrates seamlessly with other IBM analytics tools for a unified environment.
- Advanced data governance features ensure data accuracy and compliance.
- Scalable architecture handles high volumes of data and complex queries efficiently.
Considerations:
- May require familiarity with IBM technologies for optimal utilization.
- Potential vendor lock-in if heavily reliant on other IBM analytics services.
9. Oracle Autonomous Warehouse
Oracle Autonomous Warehouse offers self-driving data warehousing with automated management in the Oracle Cloud. It leverages machine learning for workload optimization and resource allocation, and integrates with other Oracle services.
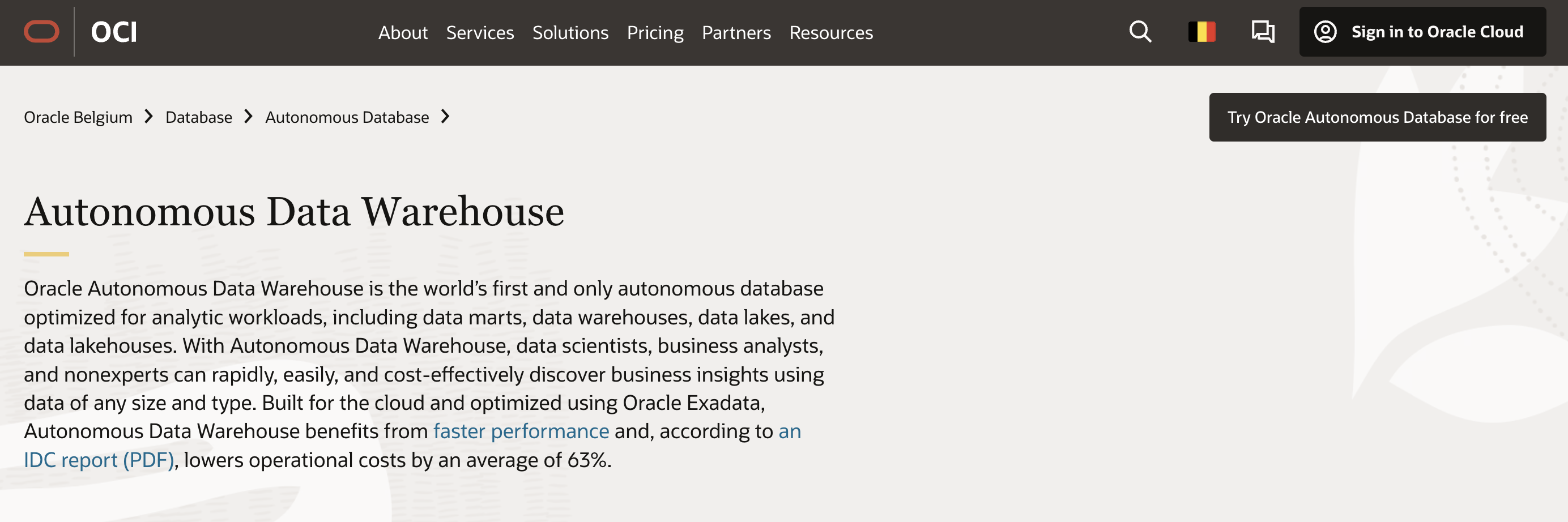
The self-driving architecture automates management tasks, simplifying data warehouse operations for organizations using the Oracle Cloud.
Features:
- Self-driving architecture automates management tasks for simplified operations.
- Leverages machine learning for workload optimization and resource allocation.
- Integrates seamlessly with other Oracle Cloud services for a unified data platform.
Considerations:
- Potential vendor lock-in if heavily reliant on other Oracle cloud services.
- Limited customization options compared to some open-source data warehouse solutions.
10. Cloudera
Cloudera is an open-source data platform offering a flexible and customizable data warehouse solution. It handles diverse data formats and sources but requires technical expertise for deployment and management.
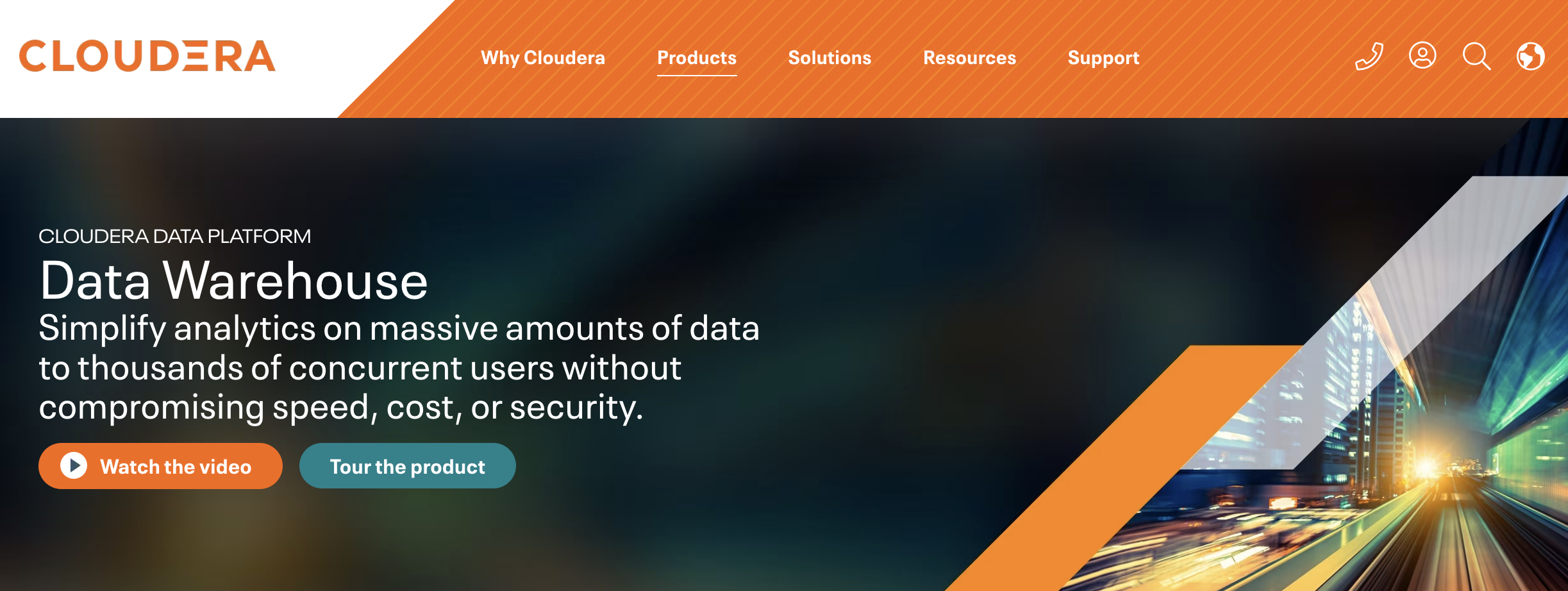
As an open-source platform, Cloudera provides greater flexibility and customization options compared to proprietary solutions.
Features:
- Open-source platform provides greater flexibility and customization options.
- Handles diverse data formats and sources for broader data integration.
- Cost-effective solution compared to some proprietary data warehouse options.
Considerations:
- Steeper learning curve compared to managed data warehouse services due to its open-source nature.
- Requires in-house technical expertise for deployment, configuration, and maintenance.
11. MarkLogic
MarkLogic is a multi-model NoSQL database that excels at handling complex data structures and relationships. It’s ideal for organizations with diverse data types and intricate data models.
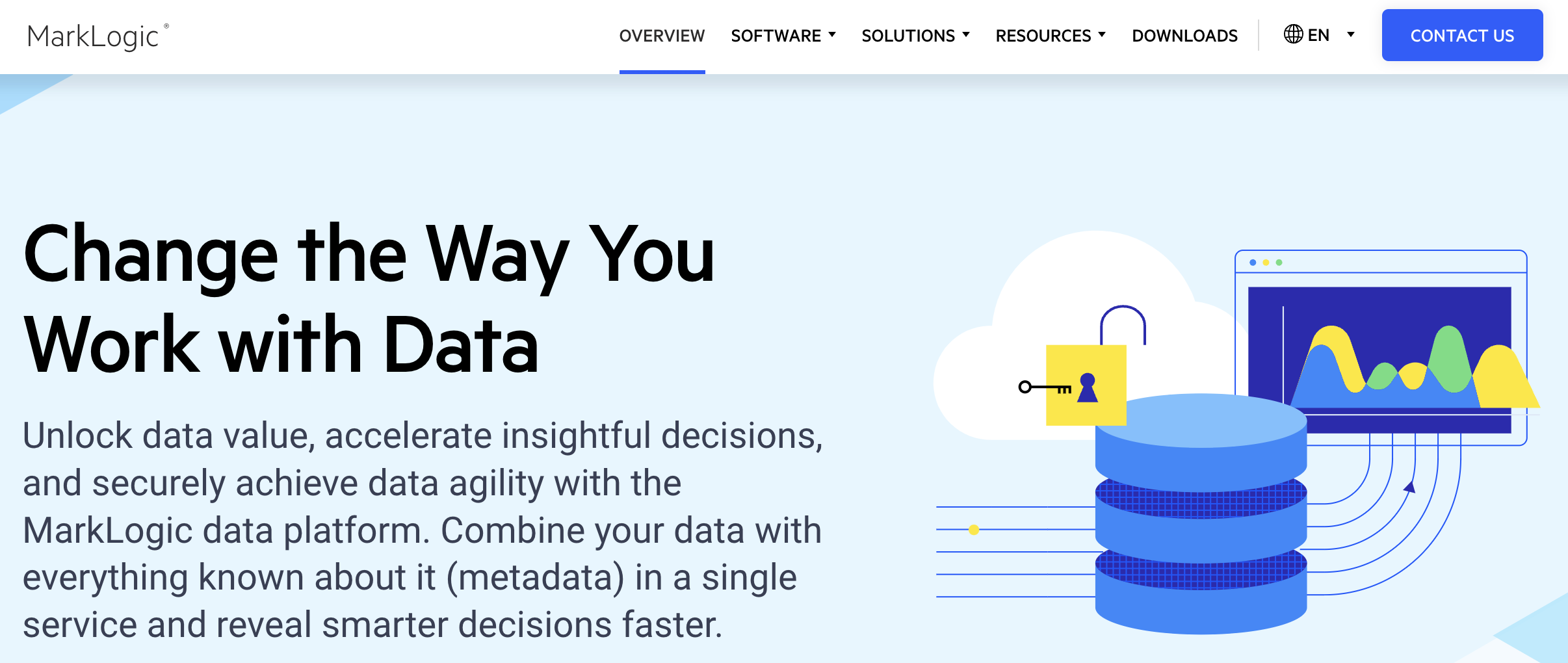
MarkLogic’s multi-model capabilities allow you to store and query structured, semi-structured, and unstructured data in a single platform.
Features:
- Multi-model NoSQL database handles structured, semi-structured, and unstructured data.
- Powerful querying capabilities for complex data exploration and analysis.
- Flexible data modeling allows for intricate relationships and hierarchies.
Considerations:
- Less familiar technology compared to traditional relational data warehouses.
- Requires specialized expertise for optimal utilization of its advanced features.
12. SAP HANA
SAP HANA is an in-memory data warehouse solution designed for real-time analytics and integration with SAP applications. It offers exceptional performance for high-speed data processing.
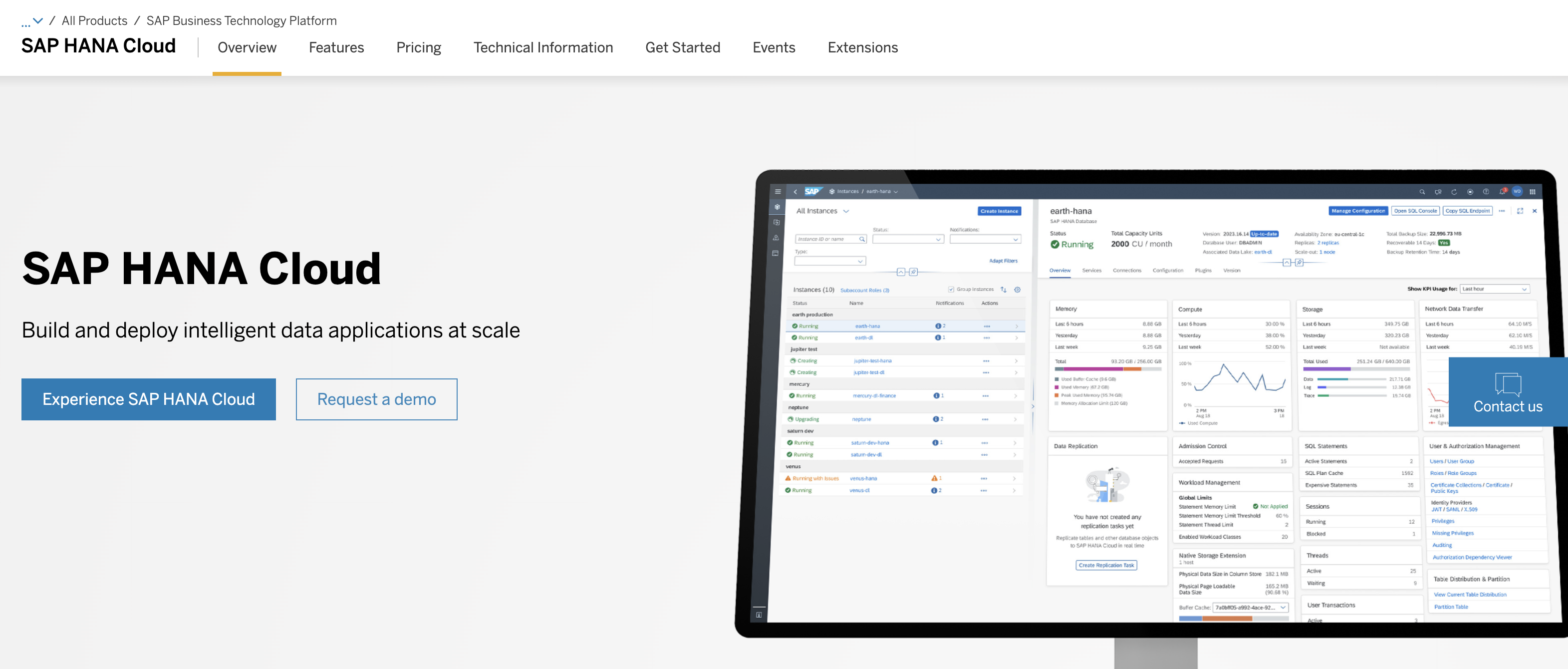
SAP HANA’s in-memory architecture enables real-time data analysis, making it a valuable tool for organizations requiring immediate insights from their data.
Features:
- In-memory architecture enables real-time analytics and data processing.
- Tight integration with SAP applications for a unified business intelligence platform.
- Optimized for handling large volumes of transaction data efficiently.
Considerations:
- Higher cost compared to some cloud-based data warehouse options.
- Primarily suited for organizations heavily invested in the SAP ecosystem.
13. Amazon DynamoDB
Amazon DynamoDB is a NoSQL database service offering high performance and scalability for various data applications, including data warehousing. It’s a good choice for real-time data workloads.
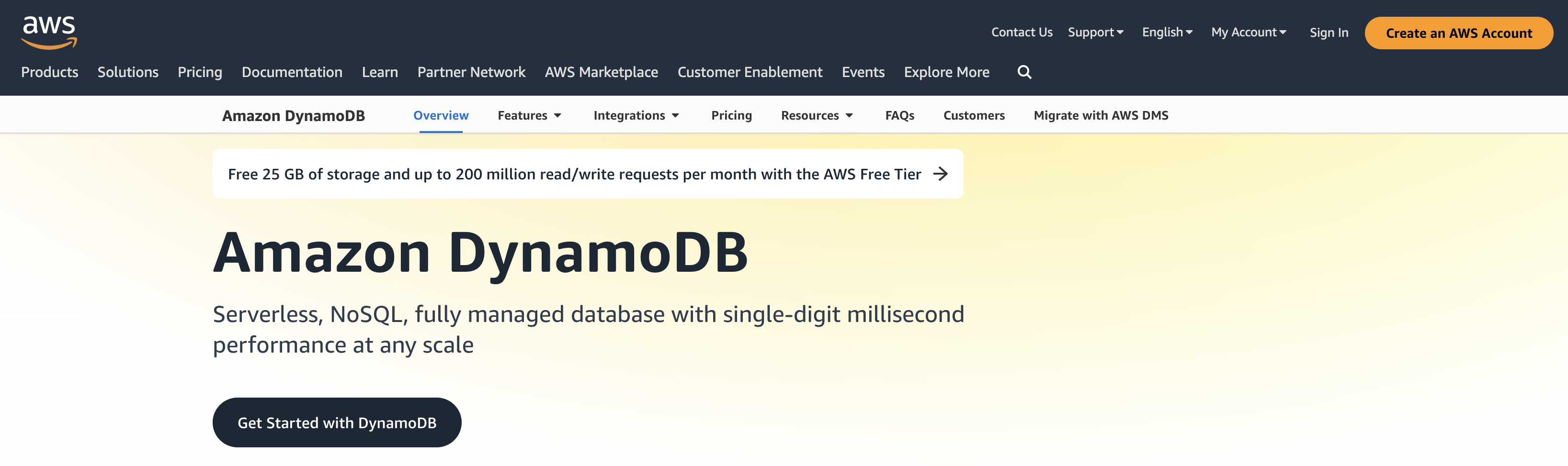
While not a traditional data warehouse solution, DynamoDB’s flexibility and scalability make it suitable for organizations with real-time data streams that require warehousing alongside other functionalities.
Features:
- NoSQL database offers high scalability and performance for diverse data workloads.
- Flexible schema design adapts to evolving data models and requirements.
- Well-suited for real-time data applications with high data velocity.
Considerations:
- Not a traditional data warehouse solution; may require additional data transformation steps.
- Might not be ideal for complex data analysis due to its lack of built-in querying features.
14. PostgreSQL
PostgreSQL is a powerful, open-source relational database management system that can also function as a data warehouse. It’s a cost-effective option for organizations comfortable with open-source technologies.
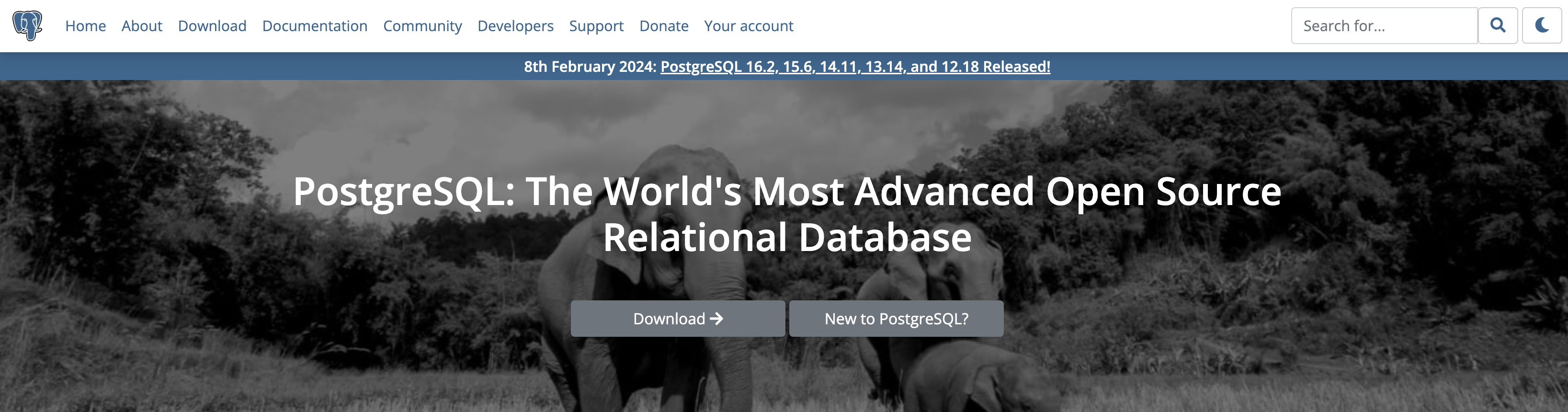
PostgreSQL offers a robust feature set for data management, querying, and security, making it a cost-effective alternative to traditional data warehouses for organizations with the in-house expertise to manage it.
Peliqan acts as a bridge, allowing you to e.g. effortlessly pull your PostgreSQL data into Google Sheets for easy access and analysis using its one-click connector. Additionally, Peliqan’s platform provides a user-friendly environment for data exploration, transformation with Magical SQL, and visualization capabilities, all without needing to switch between multiple tools.
Features:
- Open-source platform offers a cost-effective data warehousing solution.
- Robust feature set for data management, querying, and security.
- Large and active community provides extensive support and resources.
Considerations:
- Requires in-house expertise for setup, configuration, and ongoing maintenance.
- Limited scalability compared to some cloud-based data warehouse solutions.
15. MariaDB
MariaDB is another open-source relational database management system that can be used for data warehousing. It’s a robust and secure option for organizations seeking a familiar and cost-effective solution, especially those already invested in the MySQL ecosystem.
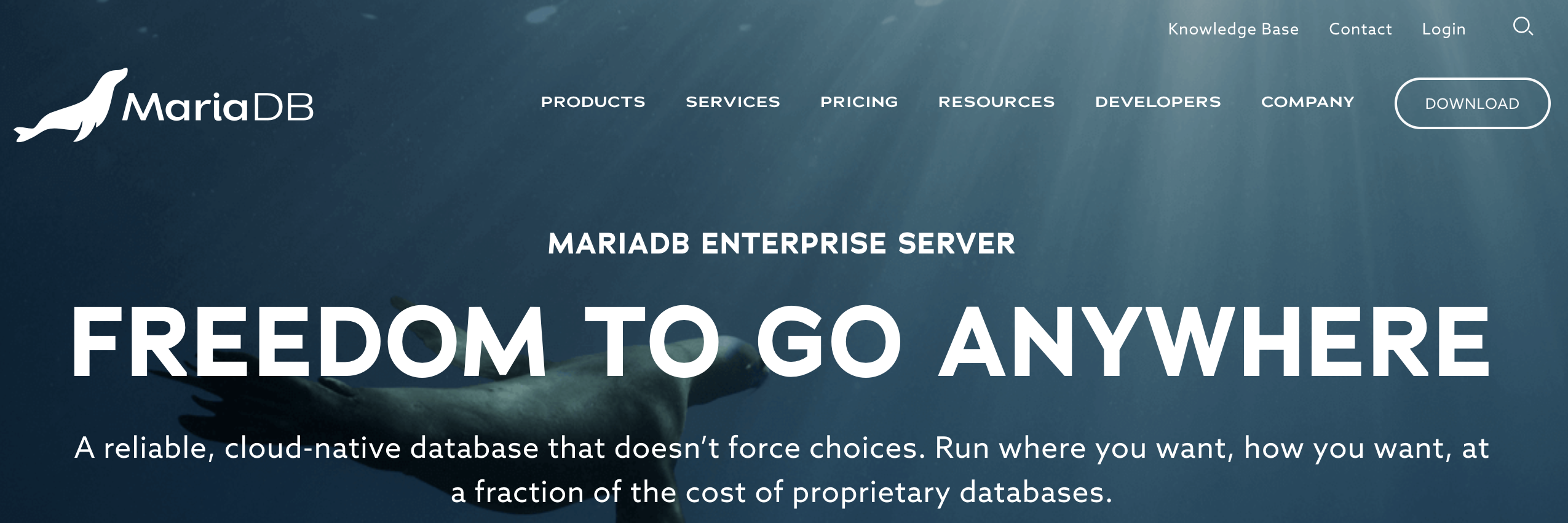
MariaDB provides a familiar SQL interface for users comfortable with relational databases, easing the learning curve for data management tasks.
Features:
- Open-source platform provides a cost-effective data warehousing solution.
- Familiar SQL interface for users comfortable with relational databases.
- High availability features ensure minimal downtime for critical operations.
Considerations:
- Requires in-house expertise for setup, configuration, and ongoing maintenance.
- Limited scalability compared to some cloud-based data warehouse solutions.
Data Warehouse Tools: Integration Capabilities
Understanding how data warehouse tools integrate with other systems is crucial for creating a cohesive data ecosystem. Here’s an overview of integration capabilities for popular tools:
Peliqan.io:
- Over 100 pre-built data connectors
- Integration with popular BI tools
- API access for custom integrations and data activation
- Built-in ETL capabilities for data transformation
- Support for real-time data streaming and batch processing
Snowflake:
- Native connectors for major BI tools (Tableau, Power BI, Looker)
- Support for various ETL/ELT tools (Talend, Informatica, Fivetran)
- REST API for custom integrations
- ODBC and JDBC drivers for broad connectivity
- Native support for semi-structured data (JSON, Avro, XML)
Google BigQuery:
- Seamless integration with Google Cloud services
- Connectors for popular BI platforms
- Support for open-source tools like Apache Beam
- BigQuery Data Transfer Service for automated data ingestion
- Integration with Google Cloud Dataflow for stream and batch processing
Microsoft Azure Synapse Analytics:
- Built-in integration with Azure services
- Power BI integration for visualization
- Support for Apache Spark
- Azure Data Factory for data integration and ETL/ELT processes
- Integration with Azure Machine Learning for advanced analytics
Amazon Redshift:
- Tight integration with AWS ecosystem
- JDBC/ODBC drivers for connecting with BI tools
- AWS Glue for ETL processes
- Amazon QuickSight for business intelligence and visualization
- Integration with Amazon S3 for data lake architecture
Micro Focus Vertica:
- ODBC, JDBC, and ADO.NET drivers for broad connectivity
- Integration with popular BI tools like Tableau and Looker
- Support for Hadoop ecosystems
- Apache Kafka integration for real-time data streaming
- R and Python integration for advanced analytics
Teradata:
- Native support for multiple BI and visualization tools
- Teradata QueryGrid for seamless data access across diverse systems
- Integration with popular ETL tools and data integration platforms
- Support for in-database machine learning with R and Python
- Teradata AppCenter for deploying and managing analytical applications
IBM Db2 Warehouse:
- Integration with IBM Cloud Pak for Data
- Support for various BI tools through JDBC and ODBC drivers
- IBM InfoSphere DataStage for ETL processes
- Integration with IBM Watson Studio for AI and machine learning
- Compatibility with open-source tools like Jupyter Notebooks
Oracle Autonomous Warehouse:
- Seamless data integration with Oracle Analytics Cloud
- Support for popular BI tools through ODBC and JDBC drivers
- Oracle Data Integrator for ETL processes
- Built-in machine learning capabilities
- Integration with Oracle Cloud Infrastructure services
Cloudera:
- Integration with various Hadoop ecosystem tools
- Support for popular BI platforms through ODBC and JDBC drivers
- Cloudera Data Engineering for ETL and data pipeline management
- Integration with machine learning frameworks like TensorFlow and PyTorch
- Cloudera DataFlow for real-time data streaming and processing
MarkLogic:
- MarkLogic Data Hub for data integration and management
- Support for BI tools through ODBC drivers
- REST and Java APIs for custom integrations
- Integration with Hadoop ecosystems
- Built-in support for semantic technologies and graph databases
SAP HANA:
- Tight integration with SAP applications and analytics tools
- SAP Data Intelligence for data orchestration and machine learning
- Support for various BI tools through ODBC and JDBC drivers
- Integration with open-source frameworks like TensorFlow and R
- SAP Analytics Cloud for business intelligence and planning
Amazon DynamoDB:
- Seamless integration with AWS services
- DynamoDB Streams for real-time data flow
- AWS Glue for ETL processes
- Integration with Amazon QuickSight for visualization
- Support for serverless applications with AWS Lambda
PostgreSQL:
- Wide range of extensions and add-ons for enhanced functionality
- ODBC and JDBC drivers for connectivity with BI tools
- Support for various programming languages (Python, Java, C/C++, etc.)
- Integration with popular ETL tools
- Foreign Data Wrappers for connecting to external data sources
MariaDB:
- MariaDB ColumnStore for analytical workloads
- Integration with popular BI and reporting tools
- Support for various programming languages and frameworks
- MariaDB MaxScale for advanced query routing and load balancing
- Compatibility with most MySQL ecosystems and tools
Data Warehouse Tools Pricing
While providing specific pricing details for all 15 data warehouse tools can be challenging due to varying configurations and usage patterns, I can offer some general insights and resources to help you estimate costs:
Cloud-Based Data Warehouses (Pricing Typically Based on Storage and Compute Usage):
- Peliqan.io: Offers flexible pricing plans based on storage and queries. Pricing starts around $150/month for basic plans.
- Snowflake: Employs a pay-per-use model for storage and compute separately. Costs can vary depending on usage, but expect to pay around $0.023 per GB per month for storage and $5 per hour for compute resources.
- Google BigQuery: Similar to Snowflake, BigQuery offers a pay-per-use model with separate charges for storage and queries. Storage costs start around $0.01 per GB per month, while on-demand queries are billed at $5 per TB processed.
- Microsoft Azure Synapse Analytics: Pricing depends on a combination of data storage, compute resources used, and additional Azure services leveraged. Costs can start around $2 per TB per month for data storage and vary based on compute usage.
- Amazon Redshift: Offers various pricing options, including on-demand instances, reserved instances, and reserved storage. On-demand pricing starts around $0.05 per hour per compute node, with storage costing around $0.023 per GB per month.
On-Premises Data Warehouses (Typically Require Upfront Licensing Costs):
- Micro Focus Vertica: Pricing varies based on server configuration and features required. Expect to pay tens of thousands of dollars for licensing fees.
- Teradata: Known for its high cost, Teradata requires contacting the vendor for a quote based on your specific needs. Expect a significant upfront investment.
- IBM Db2 Warehouse: Similar to Teradata, Db2 Warehouse pricing requires contacting IBM for a customized quote based on your deployment size and features needed.
Open-Source Data Warehouses (Free to Download and Use, But Require Infrastructure Costs):
- Cloudera: Provides a free community edition, but enterprise editions with additional features require licensing fees. You’ll also incur costs for infrastructure to run the platform.
- PostgreSQL: Free to download and use, but requires server infrastructure and technical expertise for deployment and management.
- MariaDB: Another free, open-source option requiring server infrastructure and in-house technical knowledge to set up and maintain.
Additional Considerations:
- Data Integration Costs: Factor in the cost of data integration tools or services required to move data into your data warehouse.
- Support Costs: Managed services from cloud providers typically include support, while open-source options often rely on community forums or paid support contracts.
Data Warehouse Tools
|
Type
|
Key Features
|
Best For
|
Pricing Model
|
Peliqan.io |
Cloud-based |
All-in-one platform, 100+ data connectors, Magical SQL |
Small to medium businesses, rapid deployment |
Subscription-based |
Snowflake |
Cloud-based |
Scalable, separates storage and compute |
Large datasets, SQL users |
Pay-per-use |
Google BigQuery |
Cloud-based |
Serverless, built-in ML, fast queries |
Massive datasets, advanced analytics |
Pay-per-use |
Azure Synapse Analytics |
Cloud-based |
Integrated analytics, visual tools |
Azure users, comprehensive data solutions |
Usage-based |
Amazon Redshift |
Cloud-based |
AWS integration, scalable |
AWS users, large S3 datasets |
Pay-per-use |
Micro Focus Vertica |
On-premises/Cloud |
Columnar storage, advanced compression |
Complex analytical workloads |
License-based |
Teradata |
On-premises/Cloud |
Enterprise-grade, robust security |
Mission-critical deployments |
License-based |
IBM Db2 Warehouse |
On-premises/Cloud |
IBM ecosystem integration, data governance |
IBM analytics users |
License-based |
Oracle Autonomous Warehouse |
Cloud-based |
Self-driving, ML-powered optimization |
Oracle Cloud users |
Subscription-based |
Cloudera |
On-premises/Cloud |
Open-source, flexible, handles diverse data |
Customizable data solutions |
Free/Enterprise editions |
MarkLogic |
On-premises/Cloud |
Multi-model NoSQL, complex data structures |
Diverse data types, intricate data models |
License-based |
SAP HANA |
On-premises/Cloud |
In-memory, real-time analytics |
SAP application users |
License-based |
Amazon DynamoDB |
Cloud-based |
NoSQL, high scalability |
Real-time data workloads |
Pay-per-use |
PostgreSQL |
On-premises/Cloud |
Open-source RDBMS, cost-effective |
SQL users, budget-conscious |
Free (infrastructure costs) |
MariaDB |
On-premises/Cloud |
Open-source, MySQL compatible |
MySQL users, cost-effective solutions |
Free (infrastructure costs) |
Data Warehouse Tools: Real-World Applications
While understanding the features and integration capabilities of various data warehouse tools is crucial, it’s equally important to see how these tools are applied in real-world scenarios. Different industries and organizations leverage data warehouse solutions to address specific challenges and drive business value.
To gain a deeper understanding of how data warehouses are implemented across various sectors, we’ve compiled a comprehensive guide on data warehouse examples. This resource showcases practical applications and success stories, helping you envision how these powerful tools can be tailored to meet diverse business needs.
Explore our in-depth article on data warehouse examples to discover:
- Industry-specific use cases: Learn how sectors like retail, healthcare, finance, and manufacturing are utilizing data warehouses to gain competitive advantages.
- Success stories: Read about organizations that have successfully implemented data warehouse solutions and the tangible benefits they’ve realized.
- Innovative applications: Discover unique ways companies are leveraging data warehouses to solve complex business problems and drive innovation.
- Best practices: Gain insights into effective strategies for implementing and optimizing data warehouse solutions based on real-world experiences.
- Emerging trends: Understand how cutting-edge technologies like AI and machine learning are being integrated with data warehouses to unlock new possibilities.
By exploring these examples, you’ll be better equipped to envision how the data warehouse tools discussed in this article can be applied to your specific business context. Whether you’re just starting your data warehouse journey or looking to optimize your existing setup, these real-world examples provide valuable insights and inspiration.
Understanding both the tools available and their practical applications will empower you to make informed decisions as you build and refine your data strategy. As you continue to explore the world of data warehousing, remember that the right combination of tools and implementation strategies can unlock unprecedented insights and drive significant business growth.
Choosing the Right Data Warehouse Tool
Selecting the right data warehouse tool depends on your specific needs and priorities. Consider the following factors to guide your decision:
- Data Volume and Complexity: Evaluate your data size and intricacy. Scalable cloud-based options might be ideal for massive datasets.
- Deployment Model: Cloud-based solutions offer ease of use and scalability, while on-premises options provide greater control.
- Technical Expertise: Assess your in-house technical resources. Managed services require less expertise compared to open-source solutions.
- Budget: Cloud-based services often have pay-as-you-go models, while on-premises solutions require upfront investment.
- Security and Compliance: Ensure the tool meets your data security and regulatory compliance requirements.
By carefully evaluating these factors and exploring the strengths and considerations of each data warehouse tool, you can make an informed decision that empowers your organization to unlock the value hidden within your data.
FAQ’s
What are data warehouses?
Data warehouses are centralized repositories that store massive historical datasets from various sources. Optimized for data analysis, they enable comprehensive exploration of trends and patterns to support informed decision-making.
Why use data warehouses?
Data warehouses offer significant advantages over traditional databases for large-scale historical data analysis. They facilitate faster processing, improved data quality, and deeper insights, empowering businesses to make data-driven strategic choices.
What is data warehousing with its application and example?
Data warehousing is the process of collecting and storing large amounts of data from various sources within an organization into a centralized repository, known as a data warehouse. This data is then transformed, cleaned, and optimized for querying and analysis. Applications of data warehousing include:
- Business intelligence and reporting
- Data mining and analytics
- Decision support systems
- Customer relationship management (CRM)
- Financial analysis and forecasting
An example of data warehousing could be a retail company that collects data from various sources like point-of-sale systems, e-commerce platforms, loyalty programs, and social media. This data is then loaded into a data warehouse, where it can be analyzed to gain insights into customer behavior, sales trends, inventory management, and marketing strategies.
What are examples of a data warehouse?
Some popular data warehouse tools are Peliqan.io, Snowflake, Google BigQuery, Microsoft Azure Synapse Analytics, Amazon Redshift, Micro Focus Vertica, Teradata.
Is SQL a data warehouse?
No, SQL (Structured Query Language) is not a data warehouse itself. SQL is a programming language used for managing and querying data stored in relational database management systems (RDBMS) and data warehouses. Many data warehouse solutions, such as Peliqan, Amazon Redshift, and PostgreSQL, support SQL for querying and analyzing data within the data warehouse